
Figure 2 gives another example of measurement model – a model to measure health.

If one believes that health influences cognitive ability, then one can fit a path model using the factors – cognitive ability and health. Therefore, a structural model is actually a path model. Putting them together, we have a model in Figure 3. This model is called SEM model.
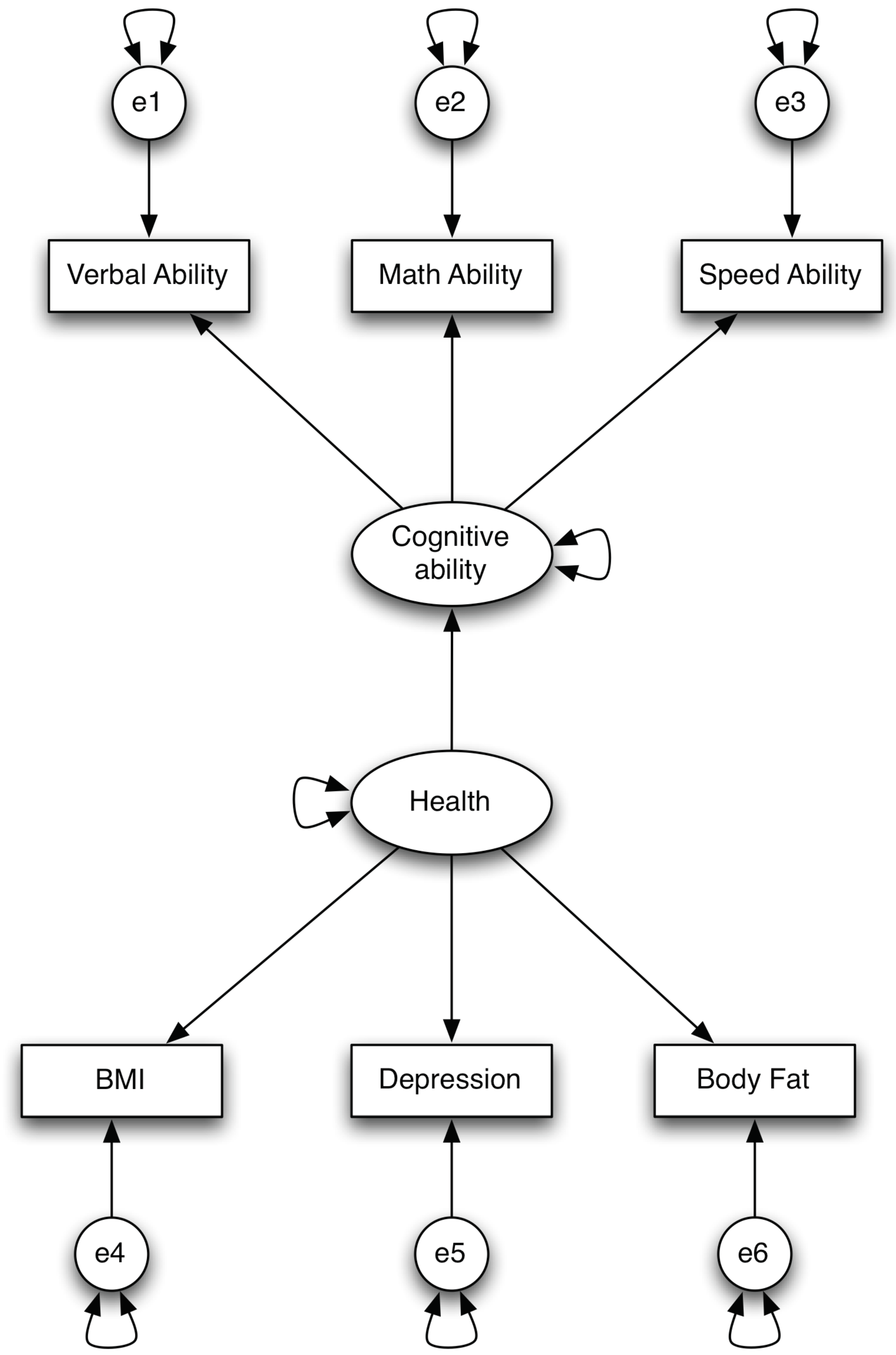
Example 1. Autoregressive model
In ACTIVE study, we have three variables – word series (ws), letter series (ls), and letter sets (ls) to measure reasoning ability. Also, we have data on all these three variables before and after training. Assume we want to test whether reasoning ability before training can predict reasoning ability after training. Then the SEM model in Figure 4 can be used. Not that we allow the factor in time 1 to predict the factor at time 2. In addition, we allow the uniqueness factors for each observed variable to be correlated. The R code for the analysis is given below.
First look at model fit. The chi-square value is 27 with 5 degrees of freedom. The p-value for chi-square test is almost 0. Thus, based on chi-square test, this is not a good model. However, CFI and TFI are both close to 1. The RMSEA is about 0.063 and SRMR is about 0.011. Considering the sample size here is large – N=1114, overall, we may accept this model is a fairly good model. Then we can answer our question. Because the regression coefficient from reasoning1
to reasoning2
is significant, reasoning ability before training seems to predict reasoning ability after training. In other words, those with higher reasoning ability before training tend to have higher reasoning ability after training.
> library(lavaan) This is lavaan 0.5-23.1097 lavaan is BETA software! Please report any bugs. > usedata('active.full.csv') > automodel <- ' + reasoning1 =~ ws1 + ls1 + lt1 + reasoning2 =~ ws2 + ls2 + lt2 + reasoning2 ~ reasoning1 + ws1 ~~ ws2 + ls1 ~~ ls2 + lt1 ~~ lt2 + ' > > auto.res <- sem(automodel, data=active.full) > summary(auto.res, fit=TRUE) lavaan (0.5-23.1097) converged normally after 66 iterations Number of observations 1114 Estimator ML Minimum Function Test Statistic 27.213 Degrees of freedom 5 P-value (Chi-square) 0.000 Model test baseline model: Minimum Function Test Statistic 5827.630 Degrees of freedom 15 P-value 0.000 User model versus baseline model: Comparative Fit Index (CFI) 0.996 Tucker-Lewis Index (TLI) 0.989 Loglikelihood and Information Criteria: Loglikelihood user model (H0) -16447.377 Loglikelihood unrestricted model (H1) -16433.771 Number of free parameters 16 Akaike (AIC) 32926.755 Bayesian (BIC) 33007.006 Sample-size adjusted Bayesian (BIC) 32956.186 Root Mean Square Error of Approximation: RMSEA 0.063 90 Percent Confidence Interval 0.041 0.087 P-value RMSEA <= 0.05 0.151 Standardized Root Mean Square Residual: SRMR 0.013 Parameter Estimates: Information Expected Standard Errors Standard Latent Variables: Estimate Std.Err z-value P(>|z|) reasoning1 =~ ws1 1.000 ls1 1.192 0.030 40.091 0.000 lt1 0.422 0.016 26.301 0.000 reasoning2 =~ ws2 1.000 ls2 1.110 0.026 43.371 0.000 lt2 0.411 0.014 29.443 0.000 Regressions: Estimate Std.Err z-value P(>|z|) reasoning2 ~ reasoning1 1.073 0.024 43.919 0.000 Covariances: Estimate Std.Err z-value P(>|z|) .ws1 ~~ .ws2 1.216 0.327 3.718 0.000 .ls1 ~~ .ls2 0.356 0.401 0.888 0.375 .lt1 ~~ .lt2 1.596 0.138 11.544 0.000 Variances: Estimate Std.Err z-value P(>|z|) .ws1 5.511 0.385 14.324 0.000 .ls1 4.547 0.475 9.575 0.000 .lt1 3.996 0.181 22.065 0.000 .ws2 5.021 0.419 11.995 0.000 .ls2 5.161 0.507 10.182 0.000 .lt2 3.963 0.181 21.875 0.000 reasoning1 19.103 1.060 18.014 0.000 .reasoning2 2.447 0.289 8.479 0.000 >
Example 2. Mediation analysis with latent variables
In path analysis, we have fitted a complex mediation model. Since we know that ws1, ls1, and lt1 are measurements of reasoning ability, we can form a latent reasoning ability variable. Thus, our mediation model can be expressed as in Figure 5.
Given CFI = 0.997, RMSEA = 0.034 and SRMR = 0.015, we accept the model as a good model even though the chi-square test is significant. Based on the Sobel test, the total indirect effect from age
to ept1
through hvltt1
and reasoning
is significant.
> library(lavaan) This is lavaan 0.5-23.1097 lavaan is BETA software! Please report any bugs. > usedata('active.full.csv') > #head(active.full) > med.model <- ' + reasoning =~ ws1 + ls1 + lt1 + reasoning ~ p4*age + p8*edu + hvltt1 ~ p2*age + p7*edu + hvltt1 ~~ reasoning + ept1 ~ p1*age + p6*edu + p3*hvltt1 + p5*reasoning + indirect := p2*p3 + p4*p5 + total := p1 + p2*p3 + p7*p3 + ' > > med.res <- sem(med.model, data=active.full) > summary(med.res, fit=TRUE) lavaan (0.5-23.1097) converged normally after 51 iterations Number of observations 1114 Estimator ML Minimum Function Test Statistic 18.363 Degrees of freedom 8 P-value (Chi-square) 0.019 Model test baseline model: Minimum Function Test Statistic 3344.605 Degrees of freedom 20 P-value 0.000 User model versus baseline model: Comparative Fit Index (CFI) 0.997 Tucker-Lewis Index (TLI) 0.992 Loglikelihood and Information Criteria: Loglikelihood user model (H0) -20675.968 Loglikelihood unrestricted model (H1) -20666.787 Number of free parameters 17 Akaike (AIC) 41385.937 Bayesian (BIC) 41471.204 Sample-size adjusted Bayesian (BIC) 41417.207 Root Mean Square Error of Approximation: RMSEA 0.034 90 Percent Confidence Interval 0.013 0.055 P-value RMSEA <= 0.05 0.889 Standardized Root Mean Square Residual: SRMR 0.015 Parameter Estimates: Information Expected Standard Errors Standard Latent Variables: Estimate Std.Err z-value P(>|z|) reasoning =~ ws1 1.000 ls1 1.181 0.029 41.262 0.000 lt1 0.430 0.016 26.758 0.000 Regressions: Estimate Std.Err z-value P(>|z|) reasoning ~ age (p4) -0.228 0.023 -9.737 0.000 edu (p8) 0.745 0.047 15.792 0.000 hvltt1 ~ age (p2) -0.161 0.027 -6.074 0.000 edu (p7) 0.429 0.052 8.177 0.000 ept1 ~ age (p1) 0.030 0.022 1.389 0.165 edu (p6) 0.396 0.046 8.527 0.000 hvltt1 (p3) 0.169 0.026 6.488 0.000 reasoning (p5) 0.643 0.035 18.186 0.000 Covariances: Estimate Std.Err z-value P(>|z|) .reasoning ~~ .hvltt1 7.255 0.592 12.263 0.000 Variances: Estimate Std.Err z-value P(>|z|) .ws1 5.334 0.366 14.594 0.000 .ls1 4.850 0.444 10.910 0.000 .lt1 3.917 0.181 21.657 0.000 .hvltt1 20.618 0.874 23.601 0.000 .ept1 11.505 0.522 22.027 0.000 .reasoning 13.814 0.777 17.777 0.000 Defined Parameters: Estimate Std.Err z-value P(>|z|) indirect -0.174 0.019 -9.368 0.000 total 0.075 0.025 3.008 0.003 >